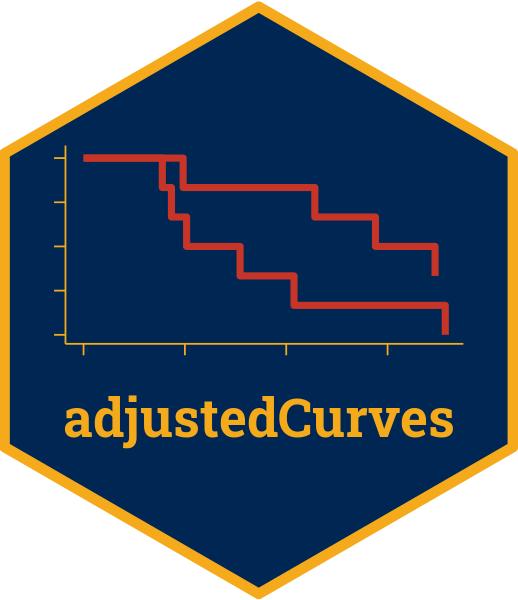
Simulate Survival Data with Confounders
sim_confounded_surv.Rd
A function to simulate time-to-event data with one or multiple confounders. The user can specify both the relationship between the covariates and the survival time and the relationship between the covariates and the treatment assignment probability. Random censoring based on a custom function may also be introduced. Can be used for simulation studies or to showcase the usage of the adjusted survival curve methodology presented in this package.
Arguments
- n
An integer specifying the sample size of the simulated data set.
- lcovars
A named list to specify covariates. Each list element should be a vector containing information on the desired covariate distribution. See details.
- outcome_betas
A named numeric vector of beta coefficients for the time-to-event outcome.
- group_beta
A number specifying the beta coefficient of the grouping variable on the survival time.
- surv_dist
A character string denoting the distribution used in the simulation of the survival time. See details.
- gamma
A numeric parameter for the simulation of the survival time. See details.
- lambda
A numeric parameter for the simulation of the survival time. See details.
- treatment_betas
A named numeric vector of beta coefficients for the treatment assignment model.
- intercept
The intercept of the treatment assignment model.
- gtol
Tolerance at which estimated treatment assignment probabilities are truncated.
- cens_fun
A function to generate censoring times or
NULL
. IfNULL
, no censoring is introduced.- cens_args
Arguments passed to
cens_fun
. Ignored ifcens_fun=NULL
.- max_t
A number denoting the maximum follow-up time. Every event time bigger than this threshold are censored.
Details
The simulation of the confounded survival data has four main steps: (1) Generation of covariates, (2) Assigning the treatment variable, (3) Generating survival times and (4) introducing censoring.
First, covariates are generated by taking independent n
random samples from the distributions defined in lcovars
.
In the second step the generated covariates are used to estimate the probability of receiving treatment (the propensity score) for each simulated person in the dataset. This is done using a logistic regression model, using the values in treatment_betas
as coefficients and interecept
as the intercept. By changing the intercept, the user can vary the proportion of cases that end up in each treatment group on average. The estimated probabilities are then used to generate the treatment variable ("group"), making the treatment assignment dependent on the covariates.
Next, survival times are generated based on the method described in Bender et al. (2005) using the causal coefficients defined in outcome_betas
and group_beta
. Both the independently generated covariates and the covariate-dependent treatment variable are used in this step. This introduces confounding.
Independent right-censoring is introduced by taking n
independent random draws from some distribution defined by cens_fun
and censoring every individual whose censoring time is smaller than its simulated survival time. The whole process is based on work from Chatton et al. (2020).
Currently only supports binary treatments and does not allow dependent censoring.
Value
Returns a data.frame
object containing the simulated covariates, the event indicator ("event"), the survival/censoring time ("time") and the group variable ("group").
References
Ralf Bender, Thomas Augustin, and Maria Blettner (2005). "Generating Survival Times to Simulate Cox Proportional Hazards Models". In: Statistics in Medicine 24.11, pp. 1713-1723
Arthur Chatton, Florent Le Borgne, Clémence Leyrat, and Yohann Foucher (2020). G-Computation and Inverse Probability Weighting for Time-To-Event Outcomes: A Comparative Study. arXiv:2006.16859v1
Examples
library(adjustedCurves)
set.seed(42)
# simulate data with default values
sim_dat <- sim_confounded_surv(n=10)
# simulate data with some new values
lcovars <- list(x1=c("rnorm", 1, 2),
x2=c("rnorm", 3, 4),
x3=c("runif", 1, 2))
treatment_betas <- c(x1=0.2, x2=0.6, x3=-0.9)
outcome_betas <- c(x1=1.1, x2=0, x3=-0.3)
sim_dat <- sim_confounded_surv(n=10, lcovars=lcovars,
treatment_betas=treatment_betas,
outcome_betas=outcome_betas)