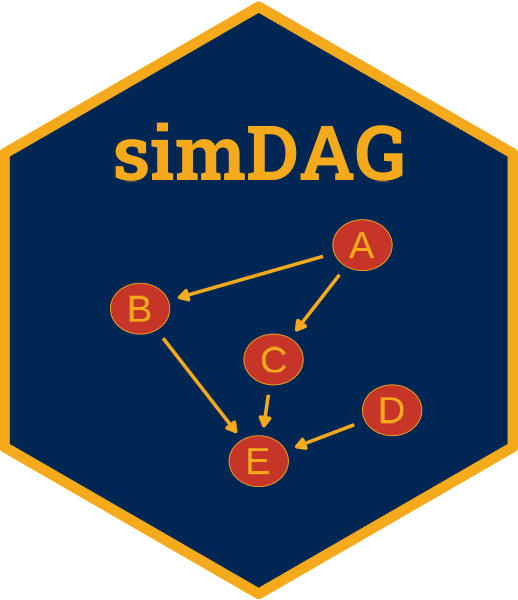
Generate multiple datasets from a single DAG
object
sim_n_datasets.Rd
This function takes a single DAG
object and generates a list of multiple datasets, possible using parallel processing
Usage
sim_n_datasets(dag, n_sim, n_repeats, n_cores=parallel::detectCores(),
data_format="raw", data_format_args=list(),
seed=stats::runif(1), progressbar=TRUE, ...)
Arguments
- dag
A
DAG
object created using theempty_dag
function with nodes added to it using the+
syntax. See?empty_dag
or?node
for more details. If thedag
contains time-varying nodes added using thenode_td
function, thesim_discrete_time
function will be used to generate the data. Otherwise, thesim_from_dag
function will be used.- n_sim
A single number specifying how many observations per dataset should be generated.
- n_repeats
A single number specifying how many datasets should be generated.
- n_cores
A single number specifying the amount of cores that should be used. If
n_cores = 1
, a simple for loop is used to generate the datasets with no parallel processing. Ifn_cores > 1
is used, the doSNOW package is used in conjunction with the doRNG package to generate the datasets in parallel. By using the doRNG package, the results are completely reproducible by setting aseed
.- data_format
An optional character string specifying the output format of the generated datasets. If
"raw"
(default), the dataset will be returned as generated by the respective data generation function. If thedag
contains time-varying nodes added using thenode_td
function and this argument is set to either"start_stop"
,"long"
or"wide"
, thesim2data
function will be called to transform the dataset into the defined format. If any other string is supplied, regardless of whether time-varying nodes are included in thedag
or not, the function with the name given in the string is called to transform the data. This can be any function. The only requirement is that it has a named argument calleddata
. Arguments to the function can be set using thedata_format_args
argument (see below).- data_format_args
An optional list of named arguments passed to the function specified by
data_format
. Set tolist()
to use no arguments. Ignored ifdata_format="raw"
.- seed
A seed for the random number generator. By supplying a value to this argument, the results will be replicable, even if parallel processing is used to generate the datasets (using
n_cores > 1
), thanks to the magic performed by the doRNG package.- progressbar
Either
TRUE
(default) orFALSE
, specifying whether a progressbar should be used. Currently only works ifn_cores > 1
, ignored otherwise.- ...
Further arguments passed to the
sim_from_dag
function (if thedag
does not contain time-varying nodes) or thesim_discrete_time
function (if thedag
contains time-varying nodes).
Details
Generating a number of datasets from a single defined dag
object is usually the first step when conducting monte-carlo simulation studies. This is simply a convenience function which automates this process using parallel processing (if specified).
Note that for more complex monte-carlo simulations this function may not be ideal, because it does not allow the user to vary aspects of the data-generation mechanism inside the main for loop, because it can only handle a single dag
. For example, if the user wants to simulate n_repeats
datasets with confounding and n_repeats
datasets without confounding, he/she has to call this function twice. This is not optimal, because setting up the clusters for parallel processing takes some processing time. If many different dag
s should be used, it would make more sense to write a single function that generates the dag
itself for each of the desired settings. This can sadly not be automated by us though.
Value
Returns a list of length n_repeats
containing datasets generated according to the supplied dag
object.
Examples
library(simDAG)
# some example DAG
dag <- empty_dag() +
node("death", type="binomial", parents=c("age", "sex"), betas=c(1, 2),
intercept=-10) +
node("age", type="rnorm", mean=10, sd=2) +
node("sex", parents="", type="rbernoulli", p=0.5) +
node("smoking", parents=c("sex", "age"), type="binomial",
betas=c(0.6, 0.2), intercept=-2)
# generate 10 datasets without parallel processing
out <- sim_n_datasets(dag, n_repeats=10, n_cores=1, n_sim=100)
if (requireNamespace("doSNOW") & requireNamespace("doRNG") &
requireNamespace("foreach")) {
# generate 10 datasets with parallel processing
out <- sim_n_datasets(dag, n_repeats=10, n_cores=2, n_sim=100)
}
#> Loading required namespace: doSNOW
#> Loading required namespace: doRNG
#>
|
| | 0%
|
|======= | 10%
|
|============== | 20%
|
|===================== | 30%
|
|============================ | 40%
|
|=================================== | 50%
|
|========================================== | 60%
|
|================================================= | 70%
|
|======================================================== | 80%
|
|=============================================================== | 90%
|
|======================================================================| 100%
# generate 10 datasets and transforming the output
# (using the sim2data function internally)
dag <- dag + node_td("CV", type="time_to_event", prob_fun=0.01)
out <- sim_n_datasets(dag, n_repeats=10, n_cores=1, n_sim=100,
max_t=20, data_format="start_stop")